CRCNS DataSet Example¶
A demonstration of Spykes’s functionality to reproduce a figure from Li et al’s “A motor cortex circuit for motor planning and movement.”
# Authors: Eric Larson <larson.eric.d@gmail.com>
#
# License: BSD (3-clause)
import os
import numpy as np
import pandas as pd
import matplotlib.pyplot as plt
import scipy.io
from spykes.plot.neurovis import NeuroVis
from spykes.config import get_data_directory
plt.style.use('seaborn-ticks')
0 Overview: Reproduce Figure¶
0.1 Article¶
Li, Nuo, et al. “A motor cortex circuit for motor planning and movement.” Nature 519.7541 (2015): 51-56. [link to paper]. We aim to reproduce this figure
0.2 Dataset¶
Nuo Li, Charles R Gerfen, Karel Svoboda (2014); Extracellular recordings from anterior lateral motor cortex (ALM) neurons of adult mice performing a tactile decision behavior. CRCNS.org [link to dataset]
1 Data¶
1.1 Download Data¶
Register in CRCNS
[link to request account]
Download file data_structure_ANM218457.tar.gz
[link]
Unzip it and you will find file data_structure_ANM218457_20131006.mat
Move this file to the right data directory.
1.2 Load Data¶
fpath = os.path.join('data_structure_ANM218457_20131006.mat')
mat = scipy.io.loadmat(fpath)
2 Get Spike Times¶
neuron_n = 9
spike_times = mat['obj']['eventSeriesHash'][0][
0]['value'][0][0][0][neuron_n - 1][0][0][1]
spike_times = [i[0] for i in spike_times]
# instantiate neuron
neuron = NeuroVis(spike_times, neuron_n)
print('neuron %d has a firing rate of %0.2f spikes per second' %
(neuron_n, neuron.firingrate))
Out:
neuron 9 has a firing rate of 5.07 spikes per second
Let’s use all the goodness of pandas
to define all our conditions.
Here, we will create a set of extra columns in the data frame that are
going to be useful to select and plot PSTHs for specific conditions. We
aim to follow the principles outlined in Hadley Wickam’s white paper on
Tidy Data.
3 Get Event Times¶
data_df = pd.DataFrame()
data_df['trialStart'] = mat['obj']['trialStartTimes'][0][0][0]
data_df['cueTimes'] = np.squeeze(
mat['obj']['trialPropertiesHash'][0][0][0][0][2][0][2])
data_df['RealCueTimes'] = data_df['trialStart'] + data_df['cueTimes']
# Collect all the events and display them
events = ['trialStart', 'cueTimes', 'RealCueTimes']
data_df[events].head()
4 Get Features¶
trialTypeMat = mat['obj']['trialTypeMat'][0][0].astype(np.bool_)
trialTypeStr = np.squeeze(
np.stack(np.squeeze(mat['obj']['trialTypeStr'][0][0])))
for ind, feat in enumerate(trialTypeStr):
data_df[str(feat)] = trialTypeMat[ind]
data_df['GoodTrials'] = np.squeeze(
mat['obj']['trialPropertiesHash'][0][0][0][0][2][0][3]).astype(np.bool_)
# Collect all features and display them
features = ['HitR', 'HitL', 'ErrR', 'ErrL', 'NoLickR',
'NoLickL', 'LickEarly', 'StimTrials', 'GoodTrials']
data_df[features].head()
5 Define Features¶
features_of_interst = ['HitR', 'HitL', 'ErrR', 'ErrL']
data_df['response'] = data_df[features_of_interst].apply(
lambda row: row.argmax() if row.max() else '', axis=1)
data_df['correct'] = data_df['response'].map(
lambda s: {'Hit': True, 'Err': False, '': np.nan}[s[:3]])
data_df['response'] = data_df['response'].map(
lambda s: {'L': 'Lick left', 'R': 'Lick right', '': ''}[s[-1:]])
data_df[['HitR', 'HitL', 'ErrR', 'ErrL', 'correct', 'response']].head()
Let’s put the events and the augmented features into a new data frame which we will use everywhere below.
# isolate trials of interest
trials_df = data_df[((data_df['GoodTrials'] == True) &
(data_df['correct'] == True))]
6 Plots¶
6.1 Rasters¶
event = 'RealCueTimes'
conditions = 'response'
window = [-3000, 2000]
rasters_fig2b1 = neuron.get_raster(event=event,
conditions=conditions,
df=trials_df,
window=window,
binsize=20,
sortby='rate',
sortorder='ascend')
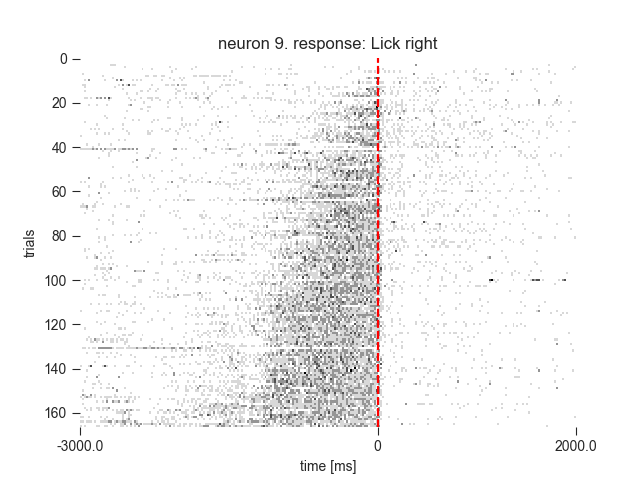
6.2 PSTH¶
plt.figure(figsize=(8, 5))
neuron.get_psth(event=event,
df=trials_df,
conditions=conditions,
window=window,
binsize=100)
plt.title('')
plt.show()
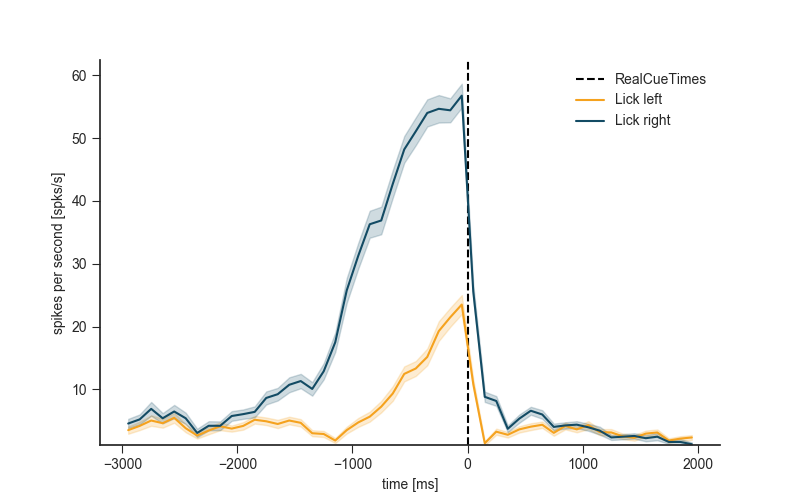
6.3 Reproduce Figure¶
plt.style.use('seaborn-ticks')
cmap = [plt.get_cmap('Blues'), plt.get_cmap('Reds')]
colors = ['#E82F3A', '#3B439A']
# get rasters------------------------------------------------------
rasters_fig2b1 = neuron.get_raster(event=event,
conditions=conditions,
df=trials_df,
window=window,
binsize=20,
plot=False)
plt.figure(figsize=(8, 10))
cond_ids = rasters_fig2b1['data'].keys()[::-1]
# plot rasters-------------------------------------------------------
for i, cond_id in enumerate(cond_ids):
plt.subplot(4, 1, i + 1)
neuron.plot_raster(rasters=rasters_fig2b1,
cond_id=cond_id,
cmap=cmap[i],
sortby=None,
has_title=False)
plt.xlabel('')
# plot psth-------------------------------------------------------
plt.subplot(212)
psth = neuron.get_psth(event=event,
conditions=conditions,
df=trials_df,
window=window,
binsize=100,
plot=True,
colors=colors)
plt.title('')
plt.show()
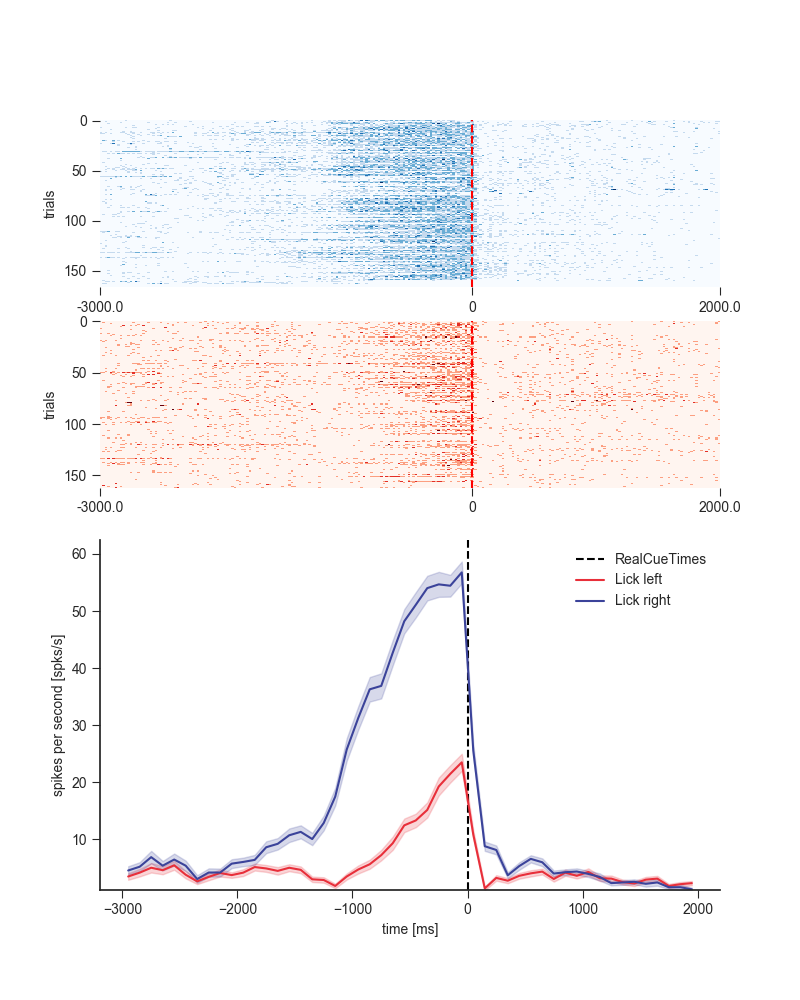
6.4 ggplot¶
plt.style.use('ggplot')
plt.figure(figsize=(8, 5))
neuron.get_psth(event=event,
conditions=conditions,
df=trials_df,
event_name='Cue On',
window=window,
conditions_names=['Lick Left', 'Lick Right'],
binsize=100)
plt.title('')
plt.show()
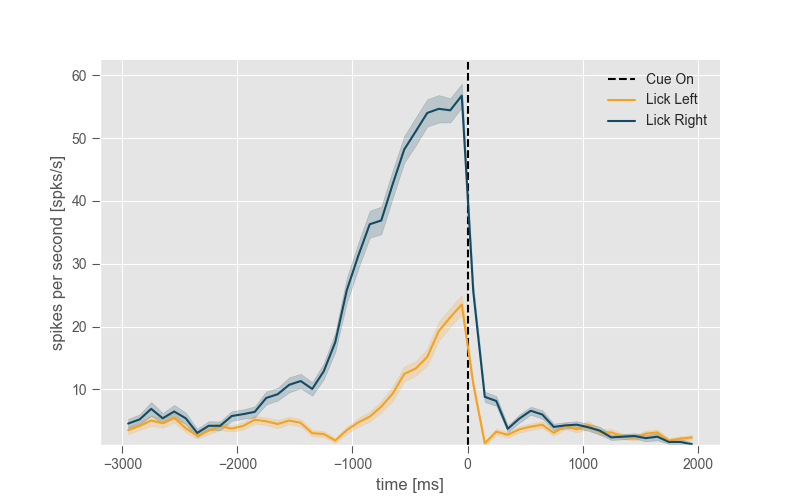
Total running time of the script: ( 0 minutes 4.161 seconds)